A Statistician Shares the Best Things About Her Field
Tian Zheng, the Statistics department chair, is developing innovative tools to confront climate change and the opioid epidemic.
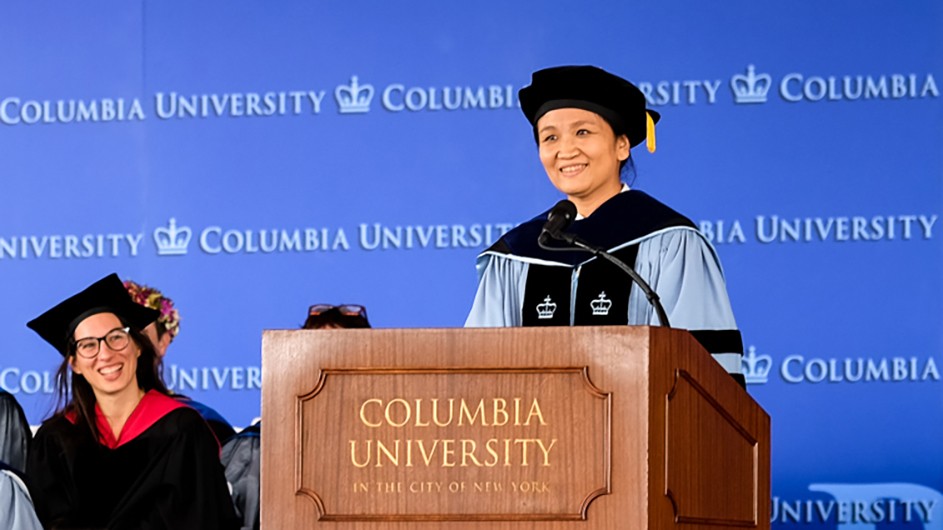
Tian Zheng first came to Columbia as a doctoral student in 1998. She joined the faculty in 2002, and became chair of the statistics department in 2019. She’s the first female professor to hold that title. This week, the American Association for the Advancement of Science named Zheng a fellow, one of the highest honors for science researchers.
On the occasion, Columbia News caught up with Zheng to discuss her ongoing work as chair of the statistics department, the projects she’s working on beyond the classroom, and how artificial intelligence is changing the field.
What’s currently under way in the statistics department?
The department is prioritizing efforts to focus on the statistical foundations of artificial intelligence, machine learning and data science, through faculty hiring, curriculum development, and collaboration with other departments, centers and institutes. Our faculty collaborate broadly across Columbia from Astronomy, Neural Science, Biology, to Political Science and Journalism. We have an innovative Applied Statistics minor that offers applied data science skills to undergraduates in any majors. One of my passions is the integration of research and education. We are introducing more research-related learning experiences into our curriculum, from undergraduate seminars, project-based learning, to mentored research and summer research experiences. AI is a topic being discussed in every Statistics department. We are no exception. How should we leverage AI in our teaching? How should we teach Statistical Thinking for a data-rich society? How can we inspire and train the next generation of statistical researchers who will work on problems important for making AI algorithms trustworthy such as algorithmic fairness, causal inference, differential privacy, and so on? How can we maximize the use of AI to accelerate scientific discovery? Those questions will define the future, so they’re top of mind for us now.
Are there any projects beyond your work as department chair that you’re particularly excited about right now?
There are a few. One is my role in Learning the Earth with Artificial Intelligence and Physics, also known as LEAP, a National Science Foundation (NSF) Science and Technology Center.
LEAP’s mission is to improve climate projection by merging physical models with machine learning. This requires better syncing the work that climate scientists and data scientists are doing. I’m one of the co-principal investigators of the group, and my job is to drive convergence between the two disciplines.
I’m also, through LEAP, working with climate scientists to develop new machine learning algorithms for studying interesting, data-intensive problems.
What’s an example of a problem you’re working on?
One that I’m working on now is a survey study of machine learning methods used in climate modeling to identify common foundations, shared workflows, and open statistical challenges. I am leading a team of statistics students in reviewing the use of machine learning in recent climate science literature. What we’re basically looking for are unified ways to translate tasks and problems in climate modeling into machine learning problems. The goal is to develop rigorous statistical frameworks and standard work flows so that we can make the deployment of machine learning in scientific research more efficient and more reproducible.
Are there any major projects you’re working on, beyond LEAP?
I’m collaborating with Nabila El-Bassel, a University Professor of social work, on an effort to use AI to better understand fatal overdose and to combat drug abuse. We’re introducing AI into her important work, building on a project she and I did together estimating the prevalence of opioid use disorder using the multiple sources of noisy and incomplete data. The most challenging and interesting aspect of this collaboration is integrating rigorous statistical research design with the power of artificial intelligence technologies to derive interpretable and actionable insights.
When did your work start incorporating machine learning?
There’s a very blurred line between machine learning, artificial intelligence and statistics. My Ph.D. dissertation was about developing innovative statistical strategies to identify important genetic variants for complex diseases. Nowadays, that would be called machine learning. At the time, it was just called statistical genetics.
Even my undergraduate thesis in Applied Mathematics was about using statistical models to analyze sound waves for a classifier of the syllables in spoken words. Nowadays we might have called that AI.
Have you always been interested in using statistics to help address social problems, like climate and the opioid epidemic?
There’s a famous saying in statistics that the best thing about being a statistician is that you get to play in everybody else’s backyard. As statisticians, we get to collaborate with all kinds of researchers and develop generally applicable research in multiple domains.
One yardstick I think is useful when I’m working on a project is: Could I explain this to a child? I thought of that a few years ago when my kids were younger, and I felt that if I could explain something to them in a way that their generation could appreciate, and understand what the use of the research was to society, it probably meant my research would have real impact.
Did you expect to stay at Columbia when you first came here in 1998?
The reason for my staying is in many ways due to the two body problem: My husband also has a PhD and there weren’t that many places where both of us could have a successful career. New York was one of them. I’m happy to be here.